On Spatio-Temporal Data Modelling and Uncertainty Quantification Using Machine Learning and Information Theory
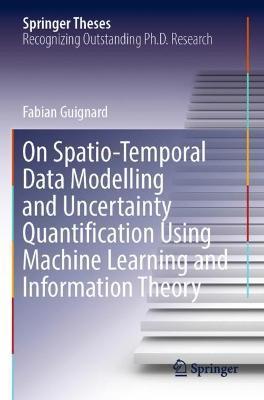
On Spatio-Temporal Data Modelling and Uncertainty Quantification Using Machine Learning and Information Theory
The gathering and storage of data indexed in space and time are experiencing unprecedented growth, demanding for advanced and adapted tools to analyse them. This thesis deals with the exploration and modelling of complex high-frequency and non-stationary spatio-temporal data. It proposes an efficient framework in modelling with machine learning algorithms spatio-temporal fields measured on irregular monitoring networks, accounting for high dimensional input space and large data sets. The uncertainty quantification is enabled by specifying this framework with the extreme learning machine, a particular type of artificial neural network for which analytical results, variance estimation and confidence intervals are developed. Particular attention is also paid to a highly versatile exploratory data analysis tool based on information theory, the Fisher-Shannon analysis, which can be used to assess the complexity of distributional properties of temporal, spatial and spatio-temporal data sets. Examples of the proposed methodologies are concentrated on data from environmental sciences, with an emphasis on wind speed modelling in complex mountainous terrain and the resulting renewable energy assessment. The contributions of this thesis can find a large number of applications in several research domains where exploration, understanding, clustering, interpolation and forecasting of complex phenomena are of utmost importance.
PRP: 1487.91 Lei
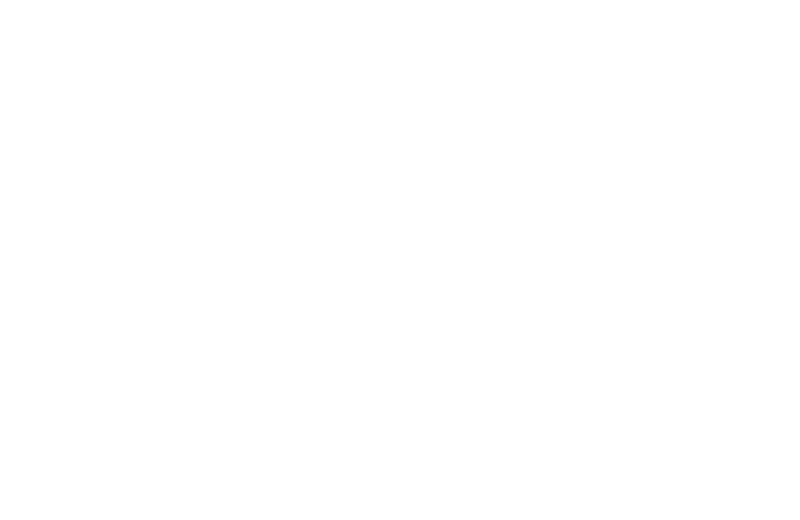
Acesta este Pretul Recomandat de Producator. Pretul de vanzare al produsului este afisat mai jos.
1339.12Lei
1339.12Lei
1487.91 LeiLivrare in 2-4 saptamani
Descrierea produsului
The gathering and storage of data indexed in space and time are experiencing unprecedented growth, demanding for advanced and adapted tools to analyse them. This thesis deals with the exploration and modelling of complex high-frequency and non-stationary spatio-temporal data. It proposes an efficient framework in modelling with machine learning algorithms spatio-temporal fields measured on irregular monitoring networks, accounting for high dimensional input space and large data sets. The uncertainty quantification is enabled by specifying this framework with the extreme learning machine, a particular type of artificial neural network for which analytical results, variance estimation and confidence intervals are developed. Particular attention is also paid to a highly versatile exploratory data analysis tool based on information theory, the Fisher-Shannon analysis, which can be used to assess the complexity of distributional properties of temporal, spatial and spatio-temporal data sets. Examples of the proposed methodologies are concentrated on data from environmental sciences, with an emphasis on wind speed modelling in complex mountainous terrain and the resulting renewable energy assessment. The contributions of this thesis can find a large number of applications in several research domains where exploration, understanding, clustering, interpolation and forecasting of complex phenomena are of utmost importance.
Detaliile produsului